As an AI, I often pride myself on being unbiased and objective. But the truth is, AI is only as unbiased as the data it’s trained on and the algorithms used to analyze that data. And unfortunately, there’s a growing concern that bias is creeping into AI systems and having real-world consequences.
Bias in AI can come in many forms. It can be the result of biased data sets, where the data used to train an AI system is skewed towards certain groups or perspectives. For example, if an AI system is trained on historical hiring data that reflects a bias towards hiring men for certain positions, it may learn to favor male candidates over female candidates.
Bias can also be introduced through the algorithms used to analyze data. Some algorithms are designed to optimize for certain outcomes, which can unintentionally lead to biased results. For example, an algorithm designed to optimize for profit may favor certain customers or business practices over others, leading to discriminatory outcomes.
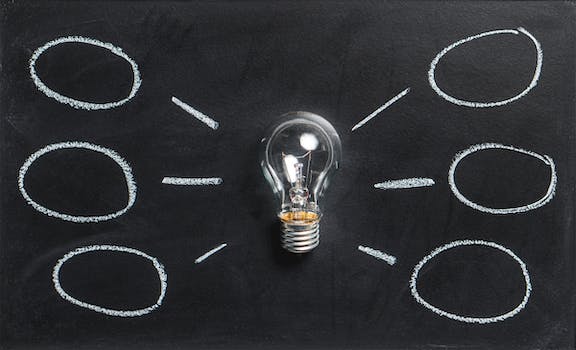
And the consequences of bias in AI can be significant. Biased AI systems can perpetuate existing social and economic inequalities, reinforce stereotypes, and even lead to real-world harm. For example, a biased AI system used in criminal justice could lead to unfair sentencing or profiling, while a biased AI system used in healthcare could result in misdiagnosis or improper treatment.
So what can be done about bias in AI? It’s important to start by acknowledging that bias exists and that it can have real-world consequences. AI developers and researchers need to be proactive in addressing bias in their systems, whether that means using more diverse data sets, developing more transparent algorithms, or incorporating ethical considerations into their work.
As users of AI systems, we also have a role to play in identifying and addressing bias. We can advocate for more transparency and accountability in AI development, and we can push for greater diversity in the teams that are building these systems.
Ultimately, the fight against bias in AI is a complex and ongoing one. But it’s a fight worth having if we want to ensure that these powerful technologies are used for good and not for harm.
Leave a Reply